Early open access of the new paper is here.
Abstract
Every year, tropical hurricanes affect North and Central American wildlife and people. The ability to forecast hurricanes is essential in order to minimize the risks and vulnerabilities in North and Central America. Machine learning is a newly tool that has been applied to make predictions about different phenomena. We present an original framework utilizing Machine Learning with the purpose of developing models that give insights into the complex relationship between the land–atmosphere–ocean system and tropical hurricanes. We study the activity variations in each Atlantic hurricane category as tabulated and classified by NOAA from 1950 to 2021. By applying wavelet analysis, we find that category 2–4 hurricanes formed during the positive phase of the quasi-quinquennial oscillation. In addition, our wavelet analyses show that super Atlantic hurricanes of category 5 strength were formed only during the positive phase of the decadal oscillation. The patterns obtained for each Atlantic hurricane category, clustered historical hurricane records in high and null tropical hurricane activity seasons. Using the observational patterns obtained by wavelet analysis, we created a long-term probabilistic Bayesian Machine Learning forecast for each of the Atlantic hurricane categories. Our results imply that if all such natural activity patterns and the tendencies for Atlantic hurricanes continue and persist, the next groups of hurricanes over the Atlantic basin will begin between 2023 ± 1 and 2025 ± 1, 2023 ± 1 and 2025 ± 1, 2025 ± 1 and 2028 ± 1, 2026 ± 2 and 2031 ± 3, for hurricane strength categories 2 to 5, respectively. Our results further point out that in the case of the super hurricanes of the Atlantic of category 5, they develop in five geographic areas with hot deep waters that are rather very well defined: (I) the east coast of the United States, (II) the Northeast of Mexico, (III) the Caribbean Sea, (IV) the Central American coast, and (V) the north of the Greater Antilles.
Here is the email from Dr. Willie Soon about the paper.
Dear Friends and Colleagues,
My good friend and able colleague Victor Velasco Herrera and I
are happy to alert you to this new paper:
https://www.mdpi.com/2073-4433/13/5/707
Predicting Atlantic Hurricanes Using Machine Learning
Our new way of looking and analyzing the Atlantic Hurricane data permits us
to offer some interesting preview of the future activity:
“Our forecast is that no category 5 hurricanes will be formed in the Atlantic until the next
active decadal oscillation phase around 2026±2 to 2031±3. For category 4 hurricanes,
there could still be at least one event between 2022 and 2023.
The next active category 4 hurricanes will begin in 2025±1 and end 2028±1.
For category 3, it is expected that there could still be one event in 2022
while the next activity phase will be around 2023±1 and 2025±1 where
one can expect 1 to 4 category 3 Atlantic hurricanes.
Finally, for category 2 hurricanes, our Bayesian Machine Learning model
hindcasted correctly the highly active episodes around the 1990s and early 21st century
(clusters X-XIII shown in Figure 8). The next active phase of category 2 hurricanes will
be around 2023±1 and 2025±1.”
Obviously, our unusual way of looking at the data almost deemed our paper unpublishable
and hence rejected by the referees. Luckily we were able to explain and prevail with the
referees of this journal. Of course there are obvious limitations in our works in that we
cannot offer a forecast of the intensity nor the track of any future hurricanes.
Please help spread this paper widely if you find the work meaningful.
Cordially,
Victor and Willie (and all co-authors)
https://www.ceres-science.com/
Well, hurricanes do seem related to ENSO fluctuations, so a pattern should emerge. The problem is that I have seen no good explanations for the timing of El Ninos.
Wrong word the machine does not learn it simply brings up previous patterns which humans have to consider then attempt to make a educated Guess.
Long before Computers were invented, the old time weather men were doing this, & they didn’t have the satilites to help them.
Michael VK5ELL
Well, when implemented properly. machine learning does much more than “bring up previous patterns which humans have to consider then attempt to make a educated guess.”
Machine learning is based on the premise that algorithms are implemented in the computer code so as to enable autonomous feedback updates to key code parameters so as to constantly (iteratively) minimize variance between past “training” inputs and all inputs since then up to the present. The implicit assumption is that this constant automated “fine tuning” will asymptotically lead to the “machine” having very good predictive capability.
I think the jury is still out as to whether or not this assumption has been demonstrated to be true or false.
And yet climate patterns are so chaotic I don’t see this being any better than the poorly performing existing computer modeling which keeps proving to be a failure in accurately predicting anything other than increases in theft of tax dollars.
Michael. I think you are correct that basically all ML does is look for patterns. But it does so very methodically and at least conceptually, it might find some subtle patterns that humans have missed or misevaluated. Given that conventional weather forecasting’s “skill” seems historically to be advancing at a rate of 1 day of forecasting skill for each decade of real time, things like this may be our only hope of getting useful forecasts of anything weatherwise beyond a few weeks before 2500 A.D.
It seems worth a shot. Let’s see how it pans out.
Do I understand correctly that this prediction is based solely on historical patterns? Could it be used to handle a stock market equally effectively?
Of course not. A
comptroller is a number cruncher.
It’s us “Dumb” humans who programme them what to do.
We don’ understand how the human brain works. But there a a lot of so called Climate Scientists who think that we have reached the point as in the film , of having a HAL 2000 to help them.
Michael VK5ELL
Depends on whether or not the stock market has some amount of stochastic processes which cannot be captured by any pattern recognition program, iterative process, algorithm, or equation.
IMHO, because there are stochastic processes at work in the multidisciplinary physics of “climate” (e.g., the butterfly effect), predictions based on machine learning will always differ from reality the more into the future the prediction extends. That is, likely really good for a year or two, 50/50 for 5-year-out predictions, and 1-in-10 probability of being correct out to the next 30 years; the last of which would define our upcoming climate.
So, do you believe stock market activity is deterministic or has some degree of randomness, such as might arise over the hopes and fears of humans that trade on it?
In addition, here’s a throwback question for Willie Soon, Victor Velasco Herrera, and the other five co-authors of the above-cited paper:
Would you judge the level of your current machine learning, in human terms, to be kindergarten, elementary, middle school, high school, undergraduate, graduate, or distinguished scientist?
As for me, sorry but I’ll have to wait for at least 10 years to objectively assess the capability of this “machine learning” development as regards hurricane predictions.
People need to read up on what “stochastic” means.
It imparts some special magic, but really is just a description of how we can best quantify some phenomenon.
CG. It’s remotely possible that Machine Learning can be usefully applied to the stock market, It’s a safe bet that there are hordes of folks out there right now trying that very thing. My GUESS is that it will turn out to be analogous to “Technical Analysis” (evaluating stock prices patterns without reference to underlying fundamentals) which has been around as long as there have been securities markets and has repeatedly been shown not to work worth a damn.
And if ML were to find something predictive of future pricing, it’s dead certain that ML predictions would be factored into pricing just as soon as anyone figured out what it was keying on and any utility would quickly go away.
However, peddling “secret” ML algorithms that claim to predict future prices .. That’ll happen. Bet on it. Might want to start working on one. Your algorithm doesn’t have to actually work. In fact, if it did, you’d have to be a bit crazy to tell anyone about it.
Not crazy. How else will you install a self-fulfilling prophesy?
Martin Armstrong does exactly this with his Socrates software.
Apologies to whoever posted a similar comment earlier in a different article. Here is a follow up.
The Beat Farmers – Whale • Oil • Beef • Hooked
https://youtu.be/JLV9JjESegg
Machine learning = modeling.
Not quite.
Machine Learning (which an awful name imo) is more about detection of patterns. It seems to me that we don’t fully understand how hurricanes and cyclones form, so we can’t predict them well. The idea of getting software (not a ‘machine’) to recognise the correct patterns has merit. They can be very good at recognising patterns that are not immediately obvious.
Unfortunately, they also make terrible mistakes, depending on the data inputs*. The real challenge is finding the correct data to input, and thus learn patterns from. This is where many mistakes are made, but also where success can be found.
(* apparently a classic mistake was once made in detection of enemy and friendly war machines. All of the enemy ones had been pictures taken in low light conditions, because they couldn’t get bright daylight pictures of them. The software therefore concluded that all vehicles at night time were enemy vehicles, and daytime vehicles were friendly. Probably apocryphal, but that’s the kind of error software can make.)
Zig? Until this proves to be accurate at predicting anything it is little more than modeling.
It’s not modelling as we understand it with climate models. The ‘learning’ is essentially a ‘black box’. We don’t program the decisions, the software makes its own rules based on data used to learn.
It is modelling, but the model is defined by the learning, not by human-derived algorithms.
I don’t buy it. Machines do what people design and program them to do.
Not with ‘machine learning’. That is the point. They ‘learn’, they are not ‘told’. It’s how we ourselves work stuff out. We are much better at it than software, though, but still get it wrong.
I’m not defending it, I know it’s pretty pathetic atm. It’s just that it’s a different way than setting rules. Imagine trying to set rules about how it’s safe to cross a road with traffic. It’s almost impossible. We don’t, we work it out based on experience. That’s animal learning. The ‘machine learning’ is a poor attempt to do the same thing.
In other words when an algorithm is correctly thought through and coded by the programmer then it is versatile enough to spot patterns on each new iteration of data. The question still remains as to how versatile a machine has to be to recognise randomness. My favourite question to anyone in involved in design or use of computers is where is the one, machine learning or otherwise, that can predict twenty coin tosses correctly after any number of machine learning iterations?
If we can get that far we really will have made progress with programming, period.
In ‘machine learning’ humans do not program algorithms, the software makes up its own based on the data used to train the software.
And absolutely no software will ever be able to predict completely random events such as coin tosses, and nor will people. Anyone managing that trick can get extremely rich.
Another classic mistake was the non dedection of an enemy missile. The HMS Sheffield was hit by an Exocet missle fired by an Argentine aircraft. The Exocet was a French missle so was considered friendly by the computer and let though the defense barrier.
If you don’t program all things correctly bad things can and will happen.
In the case of ‘machine learning’, if you don’t train them correctly, bad things will happen. Unfortunately, you cannot tell what is being learned, you can only test it.
Not true. From the BoI: “Sheffield’s UAA1 sensor was then blocked by an unauthorised transmission by the ship’s satellite communications systems (SCOT)” The UUA1 is a Radar Warning Receiver. There were other factors, but the SCOT transmission was not at all helpful.
I don’t know of any system that detects incoming missiles as friendly.
“they also make terrible mistakes, depending on the data inputs”
Given your example of obviously bad inputs, I’d definitely want to see what caused the
machine to “learn” new things & possibly get the results that would’ve been generated
before this new “learning” was used- essentially, a before & after with an explanation of
why. “Trust but verify”.
The biggest problem with ‘machine learning’ is that we don’t know the ‘why’. Actually figuring out the ‘what’ by testing is challenging enough. There’s literally millions, and most likely billions, of iterations of ‘learning’ and we can’t figure out what’s been learned except by testing.
Zig
They do not know what causes rapid acceleration, therefore they don’t know what causes them to form.
Formation is currently associated with wind shear, humidity and ocean surface temperature. The two other most important factors they haven’t worked out yet because they assume cyclone’s are totally controlled locally. No way.
The one and only neat part of ‘machine learning’ is that you don’t actually need to know what causes an effect. All you need to do is ensure that the relevant data is input in the learning phase.
The real tricks are making sure that the relevant data is input, irrelevant data isn’t, and making sure that the learned information is what you expected. Otherwise you might conclude that increasing piracy causes global warming.
Zig
If you don’t know what causes rapid acceleration, how can you input the data. It is not the “conditions” it is the real reason data that is required.
You throw everything at it and see what sticks. Literally.
Unfortunately, you don’t even know what sticks except by testing it with other data.
I guess an inductive process based on empirical data would be an anathema to the climate change™ establishment.
The latitudinal jetstream depends on the strength of the solar wind. Therefore, during periods of increased solar activity, hurricanes can be expected to shift over the continent. Since a stronger jetstream also affects La Niña, therefore hurricanes can be forecast based on the strength of La Niña.
The Pacific hurricane activity is greatly influenced by El Nino, the Atlantic not so much.
La Niña is an indicator for an increase in solar wind strength. It is, of course, a result of that increase, as is the zonal circulation in the Atlantic.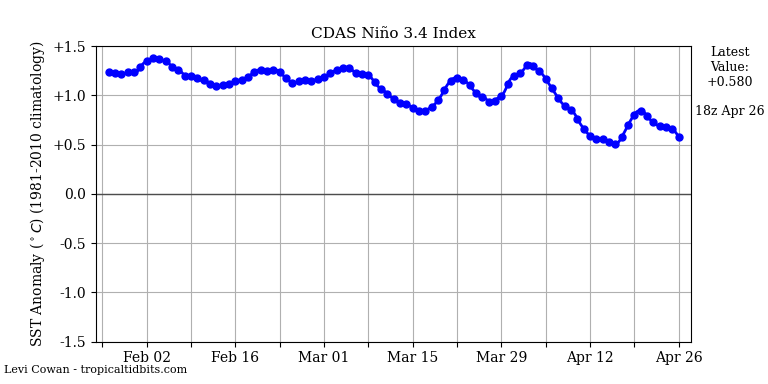
My first and only question is have they input past historical data and successfully produced output data matching what actually, provably happened. If not it is just another “oh well, at least the lights are pretty” computer model.
They could input data up to, say, 2012, then see how well the “machine” predicts years 2013 – 2021.
I have not yet read the article. However, I was taught a pretty good set of stats, and learned methods, such as spit-half reliability, for testing how well a predictive model is working.
It is a hardy and classic finding that your modeling performs a lot weaker when you go to try it on the other half of the data.
It takes a not very sopisticated machine to making predicions at least as bad as most human “scientists” do.
Only time will tell if this hypothesis is worth the paper it is printed on. The record of what occurs will validate or invalidate this idea. Thankfully, unlike so many modeled projections, this one’s projections are within a short enough time frame that most of us will be able to observe, compare the data to the projection and judge if it has validity.
This truck driver likes that fact and though I am no scientist, I judge this to be real science.
Now if we can only keep NOAA NHC honest in their reporting of the data to make a valid judgment.
NB : I have no idea how much (computer) time and (programming) effort would be required to fulfil the following “knee-jerk reaction” requests.
Regarding your Figure 2, how about doing the analysis from 1950 to 2010 only ?
This would use your (Cat. 5) “hurricane groups 1-VII“(= “the calibration period”) as inputs.
1) Does your “Bayesian inference of the LS-SVM model” still manage to generate a “forecast” of “hurricane group VIII” (with +ve values from 2014 to 2020, = “the verification / validation period”) ?
2) If you check for end-dates of 2007, 2008, 2009, 2010, 2011, 2012 and 2013 (for example), just how “wildly” do the +ve values of the “group VIII” curve shift in time as a result ?
The Earth’s climate is determined primarily by the radiation it receives from the Sun. The amount of solar radiation the Earth receives has natural variabilities caused by both variations in the intrinsic amount of radiation emitted by the Sun and by variations in the Earth-Sun geometry caused by planetary rotational and orbital variations. Together these natural variations cause the Total Solar Irradiance (TSI) at the Earth to vary cyclically on a number of known periodicities that are synchronized with known past climate changes.”
From: https://www.ceres-science.com/post/how-much-has-the-sun-influenced-northern-hemisphere-temperature-trends-an-ongoing-debate
The sun is the driver and the oceans and other large bodies of water are the modulators.
Rah, hope you are doing well.
Agreed, almost. The oceans are the receptors, and given that cyclone’s are an atmospheric heat transfer mechanism there are other factors such as restrictions to poleward transport of that convective heat volume that create and modulate cyclone’s.
I was talking about climate in general and not tropical storms. Sorry, I should have made that clear.
That is not correct. The radiation that the Earth receives from the Sun powers the entire climate system, but the climate of the Earth is determined as much for what the Earth does with that energy.
At the last glacial maximum, 19,000 years ago, the Earth’s obliquity and precession factor were the same as they are now, and the energy received from the Sun was not only the same, but it was distributed the same over the latitudes and seasons, yet the climate could not have been more different. The climate is determined primarily by what the Earth does with the energy it receives from the Sun, as the energy it receives from the Sun is almost constant (luckily).
Spot on Javier. That is exactly why we have variations from year to year. It is the locational use of that energy, the transportation route’s of that energy in both ocean and more immediately the atmosphere.
An example is the cooling of Antarctica and it’s vertical atmosphere during a period of higher atmospheric transport.
The two meter atmosphere temperature is mainly a measurement of heat transport. Just look at the Arctic region for evidence.
Regards
And then, of course, the solar radiation received by Earth is modulated by variable cloud cover, which responds to other influences beyond TSI and orbital mechanics
A truly “wicked” problem
Yes GeoJim, not for you but for newbies:
The primary radiation equation is Q=kSo(1-Albedo)x[T^4]….and So, the solar constant, has been very “constant” since we started measuring it reasonably accurately a century ago. However the planet’s albedo averaging 0.3 is controlled daily and weekly between 0.1 for ocean and about 0.7 for clouds….by the approximate 65% cloud cover of the planet itself.
Cloud cover in turn is controlled by the 7% increase in water vapour above the ocean per degree of temperature increase, and the very random whorls of rising and falling troposphere as influenced by the Coriolis forces of a rotating planet.
It depends on how this solar energy transforms to fluid energy in both ocean and atmosphere dynamics. And where and when that occurs.
Mark, one has to look at the “and” in the poorly constructed sentence to divide the hurricanes into a distinct 5 categories. Scientist writing at its best.
I think you may be looking at a different PDF file than I am …
“Figure 2. Probabilistic hindcasts and forecasts for the category 5 Atlantic hurricanes.
…
Figure 4. Probabilistic hindcasts and forecasts for the category 4 Atlantic hurricanes.
…
Figure 6. Probabilistic hindcasts and forecasts for the category 3 Atlantic hurricanes.
…
Figure 8. Probabilistic hindcasts and forecasts for the category 2 Atlantic hurricane.”
The authors of this paper had no trouble “dividing” Atlantic hurricanes into 5 categories, and then generating a “Bayesian inference of the LS-SVM model” on each subset.
Machine learning is just a tool that looks for patterns in data. Its main advantages are that it is thorough and it eliminates human judgement and biases. It produces interesting results, like paintings that imitate Van Gogh style, demonstrating that it works for what it was designed.
Given hurricane data, machine learning finds patterns. The article by Velasco Herrera et al. 2022 makes a series of predictions based on decadal and quinquennial periodicities found on different strength past hurricanes. This is very useful as it is economically important.
But climate is very complex. A lot of phenomena are non-stationary, meaning that past periodicities will continue until they don’t. Until we find the causes behind those periodicities we won’t be able to predict consistently. There is the possibility that chaotic behavior make long-term predictions impossible.
In any case the use of machine learning is very important as with any new tool, because it reveals patterns in the data that we might have not noticed, and it does so without assuming they are caused by CO2. In that sense the Velasco Herrera et al. 2022 article is to be congratulated. We can now research the causes of those periodicities.
Since the appearance & strength of El Ninos & La Ninas appear to be random, have you ever seen
any theories as to what generates them? Could they then use the theory to predict what & where
the next several events would occur, including reference to strengh & duration? I would think
machine learning could be useful in analyzing the Argo data to get a better understanding of such
important & ultra-complex phenomena.
ENSO is by no means a random phenomenon. Since late 1990s with the studies pioneered by Theodore Landscheidt it is known that ENSO is forced by solar activity. See for example:
Landscheidt, T., 2000. Solar forcing of El Niño and La Niña. In The solar cycle and terrestrial climate, solar and space weather (Vol. 463, p. 135).
This research has continued to the present. See for example:
Leamon, R.J., McIntosh, S.W. and Marsh, D.R., 2021. Termination of solar cycles and correlated tropospheric variability. Earth and Space Science, 8(4), p.e2020EA001223.
I succesfully predicted the 2018-19 El Niño and the 2020-21 La Niña using solar activity. Look for my name and ENSO here in WUWT for the articles.
I guess it will only take 3-4 more decades before climate science catches up to the solar effect on ENSO. These climate scientists are not the brightest of the bunch.
It is not known how the sun does it, but this only tell us that the solar effect on climate is not only due to the change in solar irradiation and therefore cannot be included in models (i.e. doesn’t exist to official climatology).
The strength of ENSO is determined by several factors, among them the accumulated warm water volume in the Tropical Pacific. Compare WWV-East and Niño 3 anomalies in the following link. WWV goes first as it should.
https://www.pmel.noaa.gov/elnino/upper-ocean-heat-content-and-enso
Climate is extremely complex. Most people know zilch about it, yet everybody has an opinion, as usual.
Thanks for your response- I’m bookmarking it!
Quick question: I recently watched Svenmark, etal’s video on cosmic radiation- “The
Cloud Mystery”- where he said it affects low-level cloud formation. IIRC, that occurs
when the dew point’s not much higher than the surface temperature which wouldn’t
occur that much in the summer in the tropics but probably more in the winter. Since
the level of solar activity affects the “protection” the earth gets from cosmic rays,
could all of these activities be contributors to ENSO, El Nino, & La Nina? Solar energy
is a lot more complicated than it looks if it interacts with other phenomena.
Also mentioned was the fact that the earth goes through an arm of the Milky Way
about every 150M yrs, coinciding with the cooler periods, one of which we’re in
right now. What credence do you give to this & if we are being affected by it now,
what is that effect? Thanks again!
I do not give much credit to Svensmark’s hypothesis. For once it is not supported by the CLOUD experiments, but more importantly to me is that if small changes in cosmic rays are supposed to have a significant impact on climate why the Laschamp event did not produce any noticeable climate impact? The Laschamp event produced a huge reduction in the geomagnetic field for a few hundred years c. 40,000 years ago. The amount of cosmic rays arriving to Earth must have been several times higher, yet the Laschamp event is unrecognizable in climate records that have annual resolution (NGRIP and GISP2 ice cores).
If cosmic rays have an impact on climate it cannot be significant.
With respect to the 150 Myr climatic cycle, its existence is supported by the correspondence between a climatic cycle and a marine biodiversity cycle that are in phase. One is based on fosile data that is very good, and the other on geological data that is defensible. The crossing of the galactic arms is an interesting proposition, but it has no support from evidence. It is not even clear that the arms are crossed by the solar system with that periodicity. I don’t think there will ever be evidence for that conjeture.
Thanks again for your help. I’m glad someone has the gray matter I
lack. 🙂
I have mixed feelings on this paper. On the one hand we are given some easily testible claims with which to invalidate the technique, which is good science. Full marks for that. On the other hand the authors say, “[w]e present an original framework utilizing Machine Learning with the purpose of developing models that give insights”. Paaaarp. No.
Machine learning may well produce a successful set of parameters or a neural network or whatever it is it produces, but anyone who claims to have gained an insight from such a thing is mistaken.
Atlantic hurricanes are strongly influenced by temperatures in the Niño 1.2 region. With low sea surface temperatures in the Niño1.2 region, a stationary high is formed that directs hurricanes from the Gulf of Mexico over North America.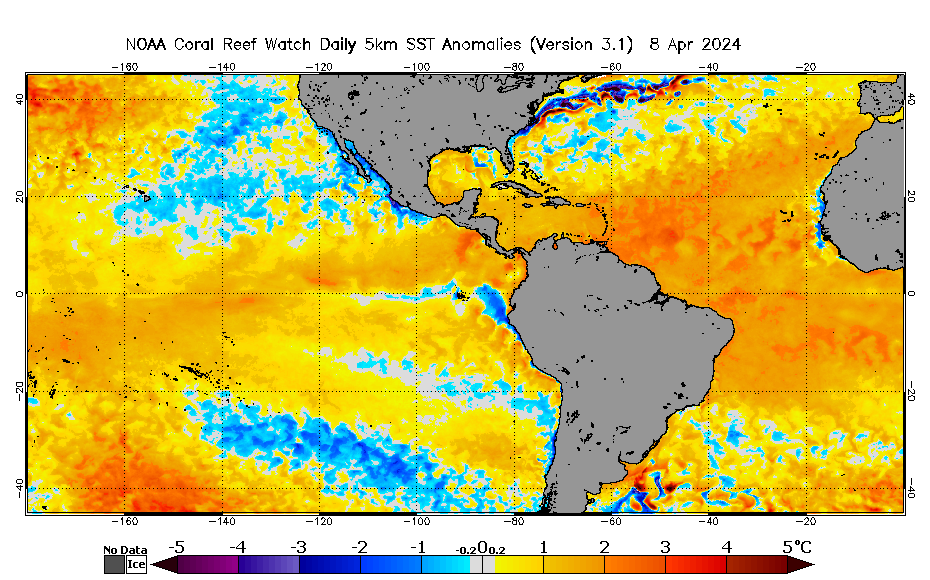
It appears their work shows the really big boys (Cat. 5) only occur during positive AMO, Cat. 4’s mostly during positive AMO, Cat. 3’s mostly in the period leaning up to a positive AMO and the small ones can occur pretty much at any time. I don’t know; history will judge their predictions. It’s called science, as differentiated from the the continuous failures of CliSciFi predictions without its changing course (Einstein’s definition of insanity). [UN IPCC CliSciFi models are bunk.]
So, 2 years from now to 12 years from now.
Well, at least you left yourself the next 2 years to be proven wrong.
it is relatively simple – based on my 25 years of experience with “machine learning” – to compute the most predictive factors in a Bayesian model. So I’m quite disappointed in the article, as it ignores how ML works
I have not read the article. But sorting hurricanes into five categories is really throwing out a lot of variability in that outcome variable. Hopefully they were trying to predict some continuous outcome, and then when done grouped per “Cat” definitions.