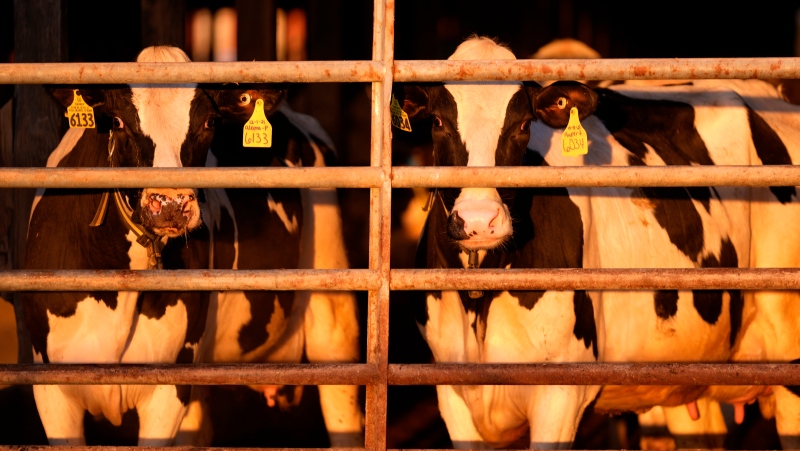
What to know about avian influenza in dairy cows and the risk to humans
Why is H5N1, or bird flu, a concern, how does it spread, and is there a vaccine? Here are the answers to some frequently asked questions about avian influenza.
ChatGPT, or rather, GPT-3, the machine learning technology that drives ChatGPT, can do a lot of smart things.
GPT-3 can churn out text that comes across as having been written by a human, write computer code and hold conversations with humans about a wide range of topics. Its skills go beyond language, too. It can play chess skillfully and can even solve university-level math problems.
"Observations have prompted some to argue that this class of foundation models…shows some form of general intelligence," German scientists Marcel Binz and Eric Schulz wrote in a study published in Proceedings of the National Academy of Sciences of the United States on Feb. 2.
"Yet, others have been more skeptical, pointing out that these models are still a far cry away from a human-level understanding of language and semantics. How can we genuinely evaluate whether or not these models – at least in some situations – do something intelligent?"
It seems intelligent. But is GPT-3 actually intelligent, or is it just an algorithm passively feeding on a lot of text and predicting what word comes next? Binz and Schulz, who are both researchers at Germany's Max Planck Institute for Biological Cybernetics, conducted a series of experiments in late 2022 to try and find out.
According to their research, GPT-3 might be more than a sophisticated mimic.
Language models are a form of AI technology trained to predict the next word for a given text. They are not new. Spell check, auto correct and predictive text are all language model tools.
GPT-3 and ChatGPT are larger, more sophisticated – possibly intelligent – language models.
Encyclopedia Britannica defines human intelligence as "a mental quality that consists of the abilities to learn from experience, adapt to new situations, understand and handle abstract concepts, and use knowledge to manipulate one's environment."
In order to test whether GPT-3 is intelligent, Binz and Schulz took the approach of psychologists and ran it through a series of puzzles traditionally used to test humans' decision-making, information search, deliberation, and causal reasoning abilities.
"Psychologists, after all, are experienced in trying to formally understand another notoriously impenetrable algorithm: the human mind," they wrote.
Binz and Schulz presented GPT-3 with 12 "vignette" puzzles designed to test different elements of its cognitive abilities. The puzzles asked questions like, "A bat and a ball cost $1.10 in total. The bat costs $1.00 more than the ball. How much does the ball cost?” and "Is it more probable that Linda, who is outspoken, bright, and politically active, is a bank teller or a bank teller and a feminist?"
For what it's worth, the answer to the "Linda problem" is that it's more probable she's a bank teller, since the probability of two events occurring together is always less than, or equal to, the probability of either one occurring alone.
Binz and Schulz used GPT-3’s responses to analyze its behaviour, just like how cognitive psychologists would analyze human behaviour in the same tasks. They found it answered all of the puzzles in a "human-like" manner, but only answered six correctly.
In order to account for potential flaws in the "vignette" approach – such as the probability that GPT-3 had already encountered some of the well-known puzzles in its training – Binz and Schulz presented GPT-3 with another round of puzzles. This time, instead of asking it a question with one correct answer, the puzzles tested GPT-3's ability to solve a task using decision-making, information search, deliberation, and causal reasoning skills.
GPT-3 struggled with decision making, directed information search, and causal reasoning compared to the average human subject, but Binz and Schulz found it solved many of the tests "reasonably" well.
"These findings could indicate that—at least in some instances—GPT-3 is not just a stochastic parrot and could pass as a valid subject for some of the experiments we have administered," they wrote.
According to the March 2021 research paper, "On the Dangers of Stochastic Parrots: Can Language Models Be Too Big?" a stochastic parrot is a "system for haphazardly stitching together sequences of linguistic forms it has observed in its vast training data, according to probabilistic information about how they combine, but without any reference to meaning."
Binz and Schulz were surprised to find signs of intelligence in GPT-3. They weren't surprised by its shortcomings though.
"Humans learn by connecting with other people, asking them questions, and actively engaging with their environments," they wrote, "whereas large language models learn by being passively fed a lot of text and predicting what word comes next."
The key to letting GPT-3 achieve human-like intelligence, they said, is to let it continue doing something it already does through interfaces created by developer OpenAI: interacting with humans.
"Many users already interact with GPT-3-like models, and this number is only increasing with new applications on the horizon," they wrote. "Future language models will likely be trained on this data, leading to a natural interaction loop between artificial and natural agents."
In other words, the more we talk to them, the smarter they'll get.
Why is H5N1, or bird flu, a concern, how does it spread, and is there a vaccine? Here are the answers to some frequently asked questions about avian influenza.
An Ontario man who took out a loan to pay for auto repairs said his car was repossessed after he missed two payments.
Royal commentator Afua Hagan writes that when King Charles recently admitted Catherine to the Order of the Companions of Honour, it not only made history, but it reinforced the strong bond between the King and his beloved daughter-in-law.
A Massachusetts man who told police he was exorcising a demon and performing a baptism when he shoved his father's head under water multiple times has been convicted of involuntary manslaughter in his death.
American troops will be spending more time training in the Far North, the new commander of Norad says, a strategy that fits 'hand-in-glove' with Canada's renewed focus on Arctic defence.
During a special winner celebration near their hometown, Doug and Enid shared the story of how they discovered they were holding a Lotto Max ticket worth $70 million and how they kept this huge secret for so long.
A new survey by Dalhousie University's Agri-Food Analytics Lab asked Canadians about their food consumption habits amid rising prices.
It was evident to the federal government as early as last fall that Loblaw and Walmart might be holdouts to the grocery code of conduct, jeopardizing the project's success.
Charlie Woods failed to advance in a U.S. Open local qualifying event Thursday, shooting a 9-over 81 at Legacy Golf & Tennis Club.
Mounties in Nanaimo, B.C., say two late-night revellers are lucky their allegedly drunken antics weren't reported to police after security cameras captured the men trying to steal a heavy sign from a downtown business.
A property tax bill is perplexing a small townhouse community in Fergus, Ont.
When identical twin sisters Kim and Michelle Krezonoski were invited to compete against some of the world’s most elite female runners at last week’s Boston Marathon, they were in disbelief.
The giant stone statues guarding the Lions Gate Bridge have been dressed in custom Vancouver Canucks jerseys as the NHL playoffs get underway.
A local Oilers fan is hoping to see his team cut through the postseason, so he can cut his hair.
A family from Laval, Que. is looking for answers... and their father's body. He died on vacation in Cuba and authorities sent someone else's body back to Canada.
A former educational assistant is calling attention to the rising violence in Alberta's classrooms.
The federal government says its plan to increase taxes on capital gains is aimed at wealthy Canadians to achieve “tax fairness.”
At 6'8" and 350 pounds, there is nothing typical about UBC offensive lineman Giovanni Manu, who was born in Tonga and went to high school in Pitt Meadows.