Interviews
Julianna Ianni, Vice President, AI Research & Development, Proscia – Interview Series
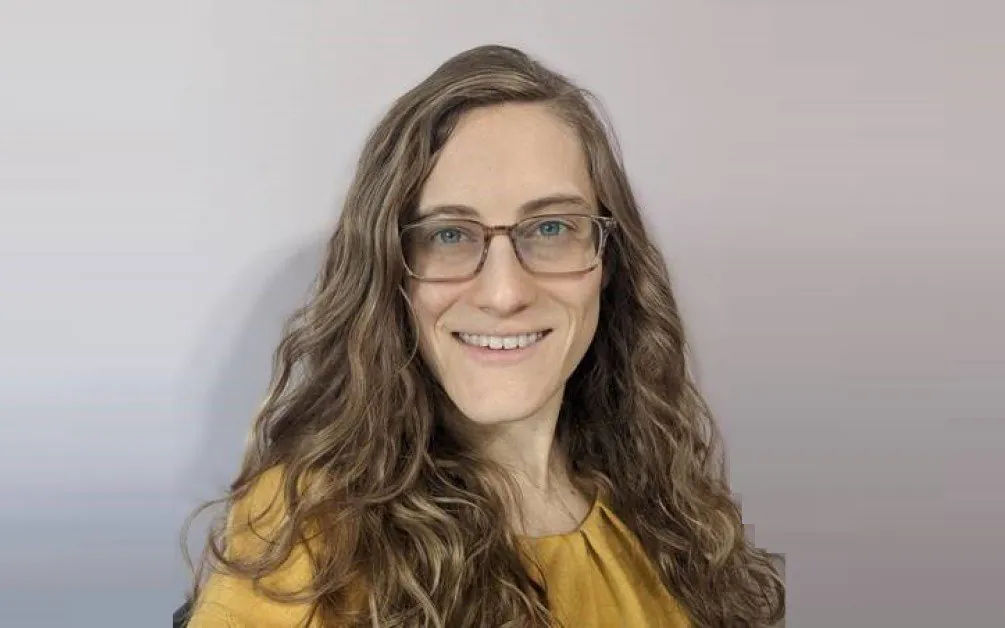
Julianna Ianni leads Proscia’s AI R&D to develop high-quality computational pathology products. Proscia is pushing labs past the limits of traditional tools with its Concentriq® digital pathology platform and pipeline of computational applications. Operating at the center of the modern digital laboratory, these technologies are transforming the economics and practice of pathology, putting the power of modern, data-centric medicine to work in the fight against cancer.
Could you share the genesis story behind Proscia?
Pathology sits at the core of biomedical research and cancer diagnosis; yet, while we’ve all seen digitization impact almost every other aspect of healthcare, pathology has remained largely unchanged over its 150-year history. It still centers around a pathologist recognizing patterns in tissue under the microscope. This practice is inherently manual and subjective, two challenges that are further compounded by a shrinking pathologist population and a rising cancer burden.
Proscia was founded to advance this standard of care. Our founders recognized an opportunity to take on the fight against cancer by driving pathology’s shift from microscope to images. In doing so – in driving digital pathology forward – we are enabling laboratories to streamline operations, achieve meaningful improvements in quality and productivity, and unlock new insights unseen by the human eye. Collectively, these benefits are helping researchers to accelerate breakthroughs and helping pathologists to improve outcomes for patients around the world.
Since our early days, we have amassed a customer base of leading laboratories, health systems, life sciences companies, and research organizations. Among our many enterprise customers, we work with Johns Hopkins University, the University of Pennsylvania, and 10 of the top 20 pharmaceutical companies. We also recently announced that LabPON, the first laboratory in the world to reach 100% digital pathology diagnosis, is transitioning to our software platform, and the Joint Pathology Center, which houses the world’s largest repository of human tissue data, is going digital with Proscia.
Proscia is a digital and computational pathology company. Can you explain to our readers what this means?
At a high level, digital pathology is the practice of digitizing glass microscope slides using a scanner, which can then be viewed, managed, shared, and analyzed using software, including computational applications leveraging AI.
Proscia specifically focuses on the software side of things. We offer a core platform, called Concentriq, that provides all of the enterprise functionality that laboratories need for carrying out their routine pathology operations. Concentriq also serves as a launchpad for AI applications, including a suite of solutions that we are building.
I highlighted many of the benefits of AI-enabled digital pathology, including driving efficiency and productivity gains and the ability to unlock new insights, above, so let’s dive into some of the specific use cases to see how these play out. One that’s especially top of mind right now is enabling remote operations during COVID. As laboratories have had to adjust to new ways of working to accommodate social distancing, digital pathology has enabled pathologists to continue working and serve patients since it enables them to easily share images and view them on-demand with a platform like Concentriq. The alternative is often for laboratories to have physical glass slides delivered to pathologists’ homes. Think about how time consuming and expensive this could be!
More generally, when we look at how digital and computational pathology solutions like those Proscia delivers are enabling pathologists to improve quality and efficiency – whether by making it easier to share images for a second opinion or by helping to eliminate error-prone, manual tasks in the lab – it is ultimately making it possible for patients to receive the right diagnosis faster, and this is important when we consider that early treatment often leads to better outcomes.
Can you describe how machine learning is being used in pathology today? Where is it going?
That’s a big question! Machine learning has really shown promise in a number of areas in pathology. One common use case for machine learning in pathology is to identify specific regions in an image where tumor tissue is located to draw a pathologist’s attention there. It can also be used to deliver quantitative insights about tissue samples – for example, counting the number of cells actively undergoing division (a common marker of cancer). Some are also working on classification problems, like being able to categorize images based on diagnosis or particular patterns that they represent, and still others are working on ways to use machine learning to predict patient outcomes or responses to specific therapies. There is so much interesting work going on in the space!
Ultimately, in pathology, most of these machine learning use cases are aimed at solving a few overarching clinical and research problems. The first is the volume problem I mentioned earlier. There is an increasing number of cases to review, and there’s this compounding issue of a decreasing number of pathologists available to perform the diagnosis of these cases. Much of machine learning in pathology aims to improve the efficiency of diagnosis at the individual and laboratory-level. The second major one is the quality of diagnosis and care – how can we improve diagnostic accuracy, how can we improve prognosis, and how can we improve patient outcomes at the end of the day?
To answer the second part of your question, I should distinguish between what’s at the research stage today, and what’s actually happening in clinical practice. Right now, most of the work in the field has been research, and it can be extremely challenging to translate some of the findings to clinical practice. That’s where I think machine learning is going and needs to go – building out the systems and the level of quality needed to actually put some of the fantastic research being done into practice, in a way that holds up to all of the amazing findings we’re seeing in the research setting and delivering these benefits to pathology labs and ultimately their patients. Building AI that works in the “real world” is and has always been Proscia’s approach.
Proscia’s DermAI leverages deep learning to pre-screen and automatically classify hundreds of variants of skin disease into pre-diagnostic categories. What were your key considerations in designing and developing this application?
First and foremost, we had to consider what we were building the system to do. We wanted it to be able to classify any skin lesion, not just a specific type. And there is quite a lot of variability in skin pathology, as you said, hundreds of variants. So, we needed to make sure we had all of that variation well-represented in our training set. This can actually be quite challenging, as some types of lesions are rarer than others, and it can be difficult to build a dataset that has enough examples of some of these rarer pathologies to train with and ensure that we had enough examples for our model to learn from.
Second, we really had to think about the fact that we weren’t just building something that needed to work at a single site, or for images scanned with a particular type of scanner. It was really about building something that could work on any laboratory’s images, on any scanner. There can be quite a lot of variation between sites and scanners in terms of the appearance of the image – colors, lightness, artifacts etc. We had to develop a system that could account for all of these variations, and not require a massive amount of data to calibrate and get it working for a new site.
We had several other considerations to account for in terms of building an AI system that could operate in the “real world.” One more, which was especially important for us, was representing the “unknowns.” After all, we know that AI systems are never perfect, and there’s so much variability in skin lesions. We needed DermAI to know what it didn’t know and be able to deliver that information when it was too uncertain to make a good classification. That’s why we built into the system a method to assign each classification a confidence score, and we took care to design this in such a way that it would be correlated with the system’s performance – the higher the confidence score, the more likely that classification is to be correct. This means we can basically tune system performance; if the way I’m using this in my lab requires extremely high accuracy, I can set DermAI to deliver high-confidence classifications only. If my use case is a little more tolerant of error and I prefer to have more cases classified, then I can include lower-confidence classifications as well.
DermAI was validated in one of pathology’s most comprehensive studies to date. Can you summarize the study and its key takeaways?
This was a study of extremely ambitious scope. As I just mentioned, there’s a tremendous amount of variability in skin lesions, which translates into their corresponding pathology images, and we wanted to develop a system that could automatically classify any routinely prepared skin pathology slide – from any lab and any scanner. The idea was that pathologists could use it to sort and triage cases before they sit down to make a diagnosis – prioritizing cases in an order that makes sense rather than in the random order they naturally come in and making sure the right cases go to the right pathologist rather than having to be sent somewhere else later. And no one had demonstrated anything close to that when we began developing this a few years ago. The system we developed broke down the task of classifying images into multiple stages using a combination of deep learning and basic computer vision techniques – detecting the tissue on the slide, adapting the image appearance so that it’s in a familiar space to the trained system, detecting the relevant regions of interest, and finally making a classification into four different categories based on the patterns present in the tissue. The important part though, was how we tested it. While we only trained the system on 5,000 images from a single site, we calibrated and tested it on almost three times as many images from three entirely separate institutions whose data our system had never seen. By doing this, we showed it was possible to build an AI application to sort and triage skin biopsies, that could perform well on multiple sites with minimal calibration. Since the data we tested on mimicked each of these sites’ prospective workload, we could be confident that the performance demonstrated here would be comparable to what we’d see if we were to install DermAI in a lab. And because the system is tunable by adjusting the confidence threshold I mentioned before, depending on what percentage of images we classify, we can tune it up to at least 98% accuracy.
One of the observations in the study was how deep learning algorithms can be sensitive to image artifacts. What precisely are these image artifacts in this case and what are some solutions to solve this problem?
Yes, a few studies have demonstrated the sensitivity of AI systems to image artifacts in pathology as in any other field. These are often simple things that our human brains overlook easily – dirt on a slide, slight changes in lighting, blurry regions of an image, pen ink that pathologists often use to mark regions of tumor. I’m listing a few examples but there are countless others. AI systems can be easily fooled by these sorts of issues if they haven’t been properly exposed to them.
There are really two routes to handling image artifacts for AI systems. The first is by cleaning – making sure that you’re training on and testing images have been meticulously cleaned, either digitally or physically, so there aren’t any artifacts present. This is sometimes easy to do for a training dataset but much harder to do consistently if you’re looking to install an AI system at many sites. So, we took the second approach: making sure that these kinds of artifacts were well represented in our data. We didn’t have slides cleaned before they were sent to us so we have a lot of representation of some of the strange issues that you might not see in a pristine training set, but that you’ll certainly ultimately encounter in the real world. This way, we could ensure that our system was ready when exposed to these artifacts in images it wasn’t trained on.
How are AI applications like DermAI being implemented in the pathology laboratory?
That’s a great question. While different laboratories are taking different approaches, we believe that the only way laboratories will truly scale their AI adoption is by leveraging an AI-enabled platform. As I described above, laboratories’ digital pathology operations center around a platform that they use for viewing, managing, and analyzing images. Proscia’s platform, Concentriq, provides all of this functionality and also serves as a launchpad for AI applications. We believe that this approach makes it easy for laboratories to deploy AI in practice, seamlessly integrating it into their day-to-day work so that they can leverage it at scale and realize its true promise.
Is there anything else that you would like to share about Proscia?
I’m really excited about the work that my team and I are doing. The unfortunate reality today is that we all know someone who has been impacted by cancer and the significant impact that it has on them and their loved ones. Our work has the potential to improve patient outcomes and truly make a meaningful difference. This is something I’m proud to be a part of.
To that end, it’s great to see that so many leading organizations also believe in the work that we are doing. Over the past few months alone, the Joint Pathology Center (JPC), which houses the world’s largest repository of human tissue data, selected Proscia to digitize this archive. JPC has several reasons for wanting to go digital, including accelerating the development of AI. LabPON, the first laboratory in the world to reach 100% digital pathology diagnosis, also recently selected Proscia’s platform to scale its pathology operations and lay the foundation for implementing AI. LabPON will also collaborate with us on the development and validation of our AI systems.
And finally, we recognize that we can’t transform the practice of pathology alone and are constantly growing our team. If you’re interested in joining us, I encourage you to learn more about Proscia and view our open roles.
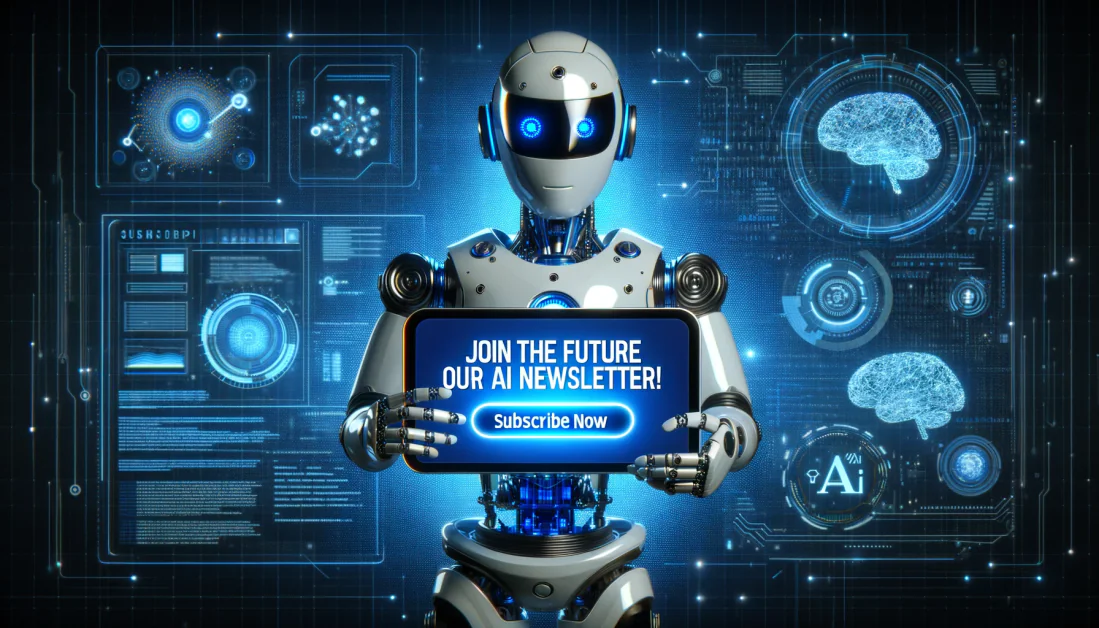
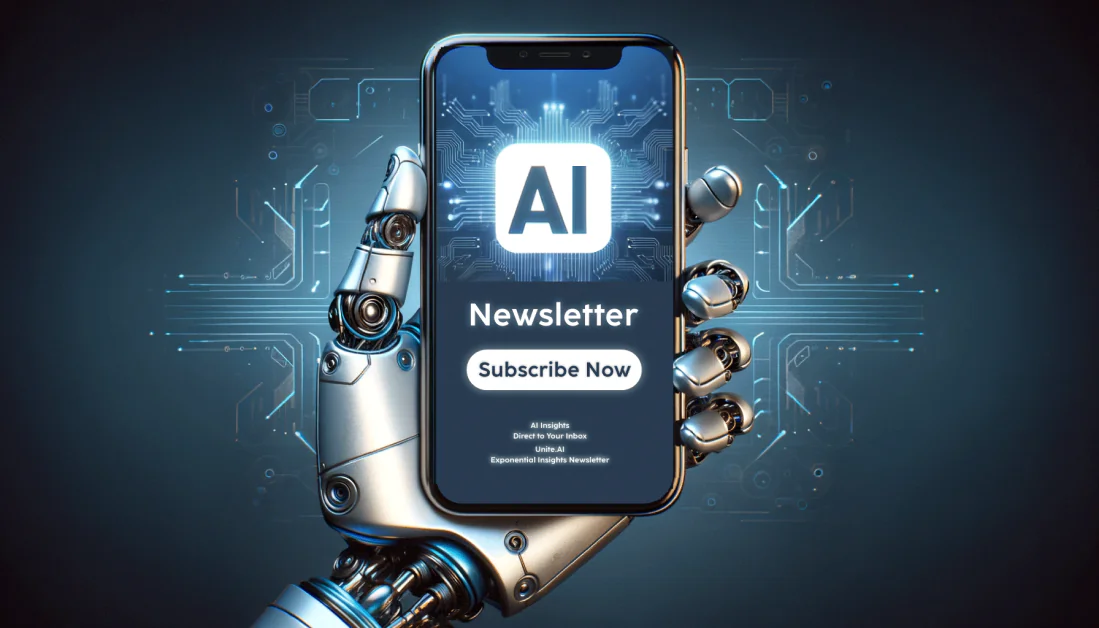